
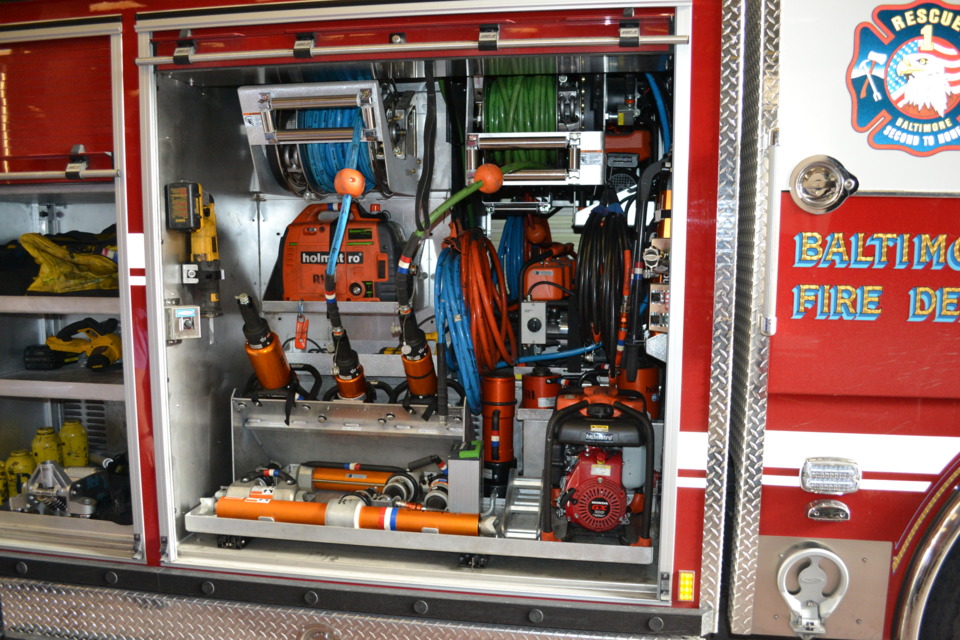
While the fundamental forms of operation and decision-making within banks have not been dramatically revolutionised, still, the question around bank operations is legitimate in the light of the emergence of AI. 1 This view exemplifies a widespread tendency to call into question the operating model of traditional banks whenever new technologies appear. Historical hindsight shows that AI is not the first technological “disruption”to have affectedthe banking industry: here it suffices to consider the rise ofautomatic teller machines and online banking.In 1990’s, Bill Gates said “banking is necessary, banks are not”. the transformation of humanity through technologically enhanced capacities (Bostrom, 2017). At the same time, it is connected to fears of technological job substitution, to the prospect ofskills retraining, to a widening digital divide and, more broadly, to a drift towards transhumanism, i.e. AI has come under public scrutiny, as part of a new mythology of the digital evoking simultaneously hope and fear.For instance, AI is credited with the prospect of a revival of consumption, of cross-sector growth in productivity, and of improved risk management, to name a few. The world of finance, too,hasn’t been left untouchedby the data science revolution sparked by artificial intelligence (AI). These limits call for the establishment of a new generation of financial regulation introducing the certification of AI algorithms and of data used by banks.Īlgorithms help guide decisions in many areas, such as medical diagnostics, predictive justice, facial recognition, fraud detection, job search, and access to higher education (ACPR, 2018). However, AI-based credit analysis processes raise enduring concerns due to potential biases and ethical, legal, and regulatory problems. On a micro scale, instead, the use of AI in credit analysis improves financial inclusion and access to credit for traditionally underserved borrowers. At the macroeconomic level, this translates into positive estimates for economic growth. Combined, the use of AI and big data can capture weak signals, whether in the form of interactions or non-linearities between explanatory variables that appear to yield prediction improvements over conventional measures of creditworthiness. The unique features of AI models, coupled with the expansion of computing power, make new sources of information (big data) available for creditworthiness assessments. This article teases out the ramifications of artificial intelligence (AI) use in the credit analysis process by banks and other financing institutions.
